The Ultimate Guide to Annotation Tools for Machine Learning
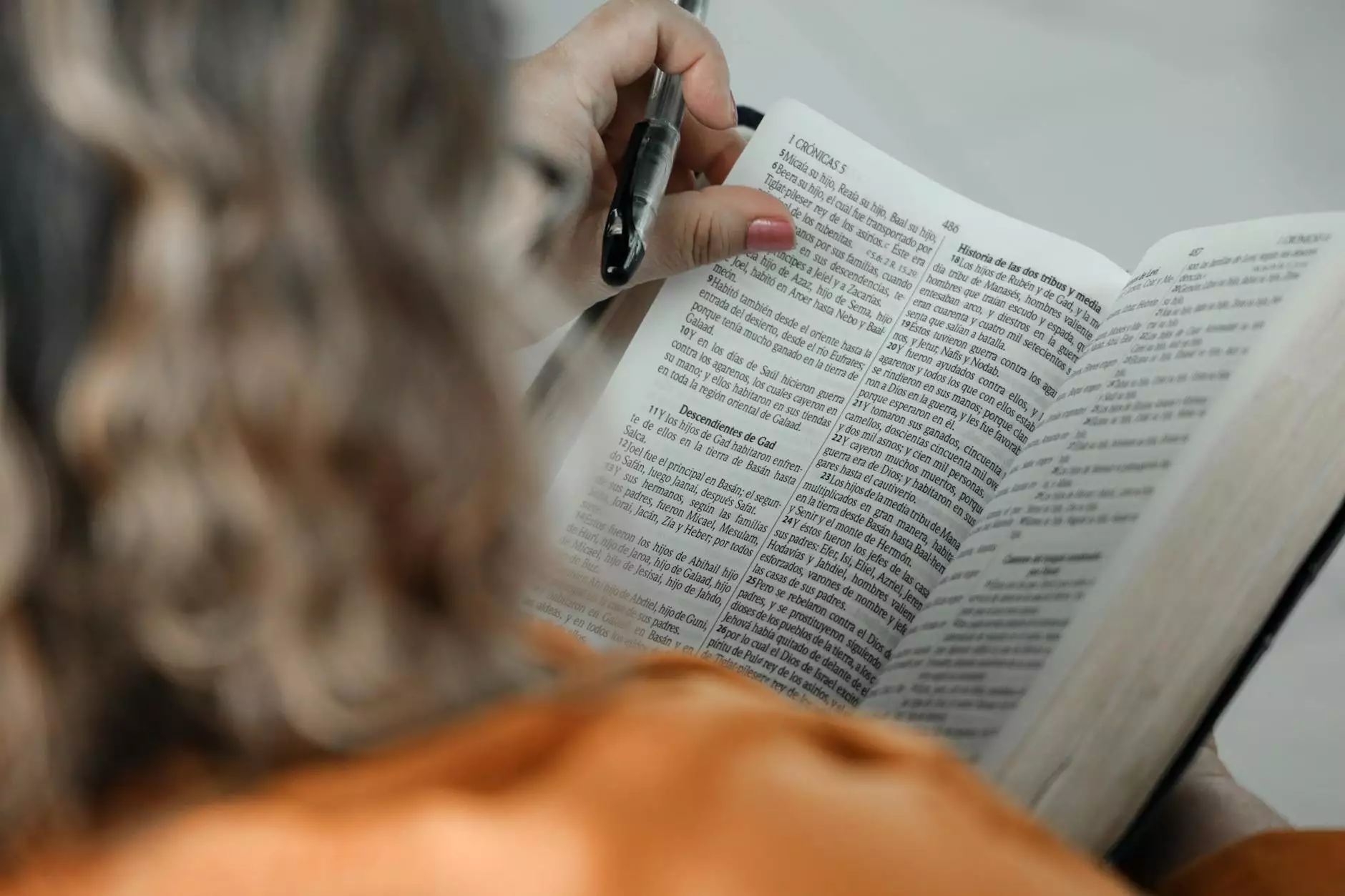
In today's world, machine learning has emerged as a revolutionary technology that reshapes industries. One of the critical components of successful machine learning applications is the quality of data used for training algorithms. This is where annotation tools for machine learning play a vital role. This article will delve deep into the significance of annotation tools, the different types available, the benefits they offer, and how they contribute to the overall machine learning ecosystem.
Understanding Data Annotation
Data annotation involves the process of labeling data to make it understandable for machine learning algorithms. The more accurately data is labeled, the better the performance of the machine learning model. Annotation tools help streamline this process, allowing data scientists and engineers to focus more on building models rather than getting caught up in data preprocessing.
Types of Data Annotation
Different machine learning tasks require specific types of data annotation. Understanding these types is crucial for selecting the right annotation tool. Here are some common types:
- Image Annotation: This includes tasks like image classification, object detection, and semantic segmentation.
- Text Annotation: Involves annotating text data for sentiment analysis, named entity recognition, and categorization.
- Audio Annotation: Used for labeling audio files for speech recognition, speaker identification, and acoustic event detection.
- Video Annotation: Involves annotating video data for object tracking, action recognition, and activity detection.
The Importance of Annotation Tools in Machine Learning
When embarking on a machine learning project, consider how annotation tools for machine learning can illuminate your path. Here are several compelling reasons:
Enhanced Accuracy
Manual annotation can lead to human errors. Automated annotation tools significantly reduce this risk, ensuring higher precision in labeling data, thus enhancing the model's overall performance. For instance, tools equipped with AI can assist in tagging images more accurately than manual efforts.
Scalability
As your data grows, so does the need for efficient annotation processes. Using appropriate annotation tools helps scale the annotation process swiftly and efficiently. Companies can handle vast datasets without compromising quality, making it easier to keep pace with the growing demand for data in machine learning.
Time Efficiency
Time is a crucial factor in any business. Efficient annotation tools minimize the time spent on data labeling, allowing teams to focus on model training and fine-tuning. Automating tedious tasks and integrating simple workflows make a significant difference in productivity.
Characteristics of Effective Annotation Tools
Choosing the right annotation tool can be daunting, but knowing what to look for can make this process easier. Here are some essential characteristics to consider:
- User-Friendly Interface: The tool should have an intuitive design that facilitates easy navigation and quick learning curves.
- Collaboration Features: Good annotation tools allow multiple users to work on projects simultaneously, enabling collaboration and coordination.
- Support for Various Data Types: The tool should support different data formats, catering to text, image, audio, and video data.
- Data Security: With the increasing focus on data privacy, selecting tools that prioritize data security is paramount.
- Integration Capabilities: Effective tools should seamlessly integrate with existing workflows and systems.
Popular Annotation Tools for Machine Learning
With the variety of annotation tools available, choosing can be overwhelming. Below we list some popular annotation tools highlighted for their robust features:
1. Labelbox
Labelbox provides a comprehensive platform for data annotation, including image and video annotation. Its collaborative capabilities, automation features, and intuitive interface make it a favorite among data scientists.
2. Snorkel
For those interested in probabilistic labeling, Snorkel offers a unique approach. It enables users to generate labels quickly without manually annotating each instance, which saves time and resources.
3. VGG Image Annotator (VIA)
VIA is an open-source image annotation tool that allows users to annotate images efficiently. It supports various formats and offers a simple interface for easy operation.
4. Supervisely
Ideal for computer vision tasks, Supervisely combines annotation, training, and deployment into one platform. Its advanced image annotation capabilities are highly regarded in the industry.
Challenges in Data Annotation
While the advantages of annotation tools are compelling, challenges persist in the data annotation process:
Quality Control
Ensuring data quality is paramount. Inaccurate labels can lead to flawed models. It's crucial to implement quality checks and balance automated processes with manual reviews.
Cost
Some annotation tools can be expensive, particularly if you're working with large datasets. It’s vital to balance quality and cost-effectiveness when choosing a tool.
Skill Requirements
Some tools require advanced knowledge to use effectively. Training and onboarding can be time-consuming. Organizations should consider the learning curve associated with any tool new to their teams.
Future Trends in Annotation Tools for Machine Learning
The landscape of annotation tools is evolving rapidly. Here are some trends that businesses like keymakr.com should keep an eye on:
- Enhanced Automation: Continued developments in AI will lead to more automation, reducing human involvement and speeding up processes.
- Improved Integration: As companies increasingly rely on various tools, improved integration capabilities will become crucial.
- Greater Focus on User Experience: More emphasis will be placed on intuitive interfaces that require minimal training.
Conclusion
In the vast realm of machine learning, data is the backbone, and annotation tools for machine learning are indispensable for ensuring that data is accurately labeled. By understanding the various types of annotation, recognizing the importance of these tools, and keeping abreast of emerging trends, businesses can harness the power of machine learning more effectively. The right annotation tools can revolutionize data processing, leading to advanced, efficient, and more accurate outcomes.
Whether your business is in Home Services, Keys & Locksmiths, or any other field, leveraging appropriate data annotation tools will not only improve your machine learning models but also keep you ahead in this competitive landscape.